Unveiling the Power of Crowdsourcing, AI Data Labeling, and E-Learning
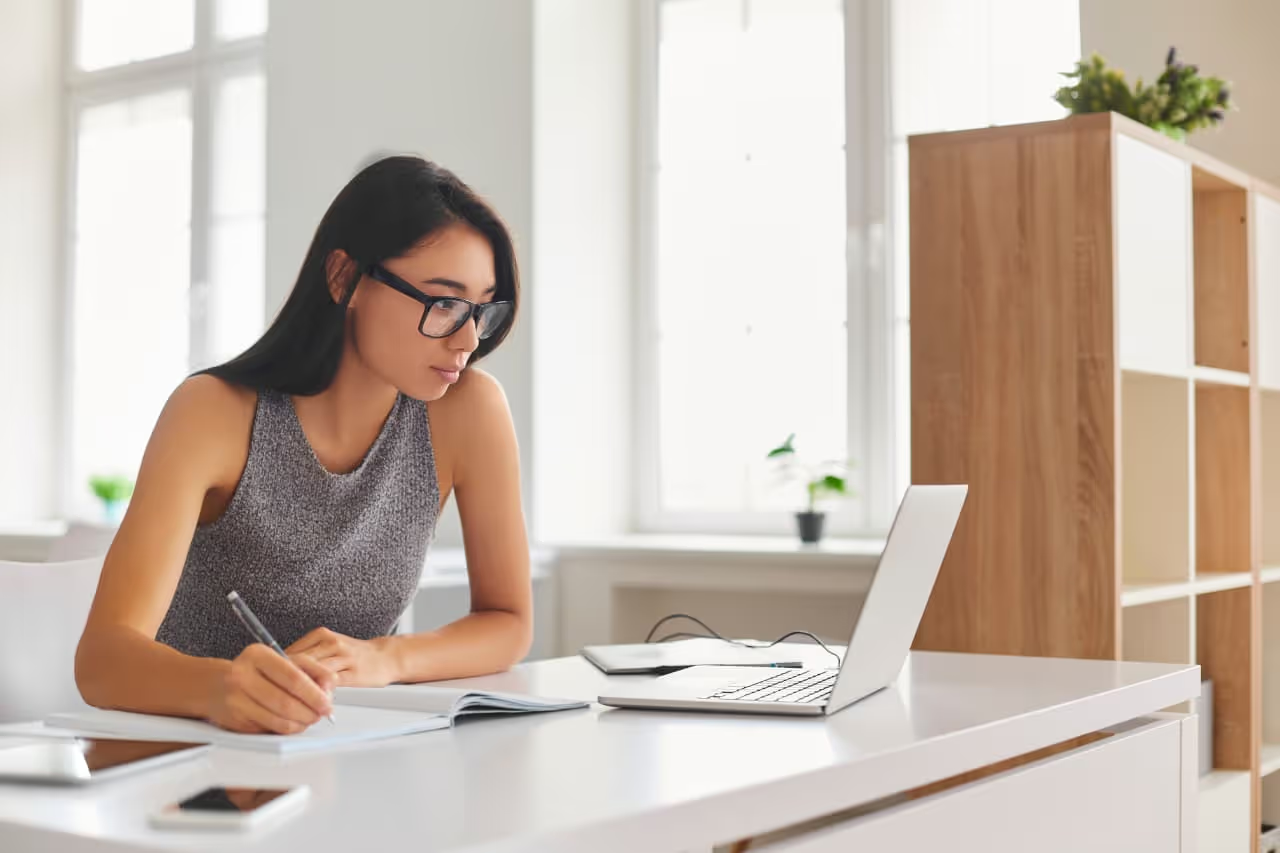
In today’s rapidly evolving digital landscape, three concepts are making waves across various industries: crowdsourcing, AI data labeling, and e-learning. Each of these has its own unique attributes and benefits, contributing to innovation and efficiency in different ways. Let’s delve deeper into what these terms mean and how they’re shaping our world.
What is Crowdsourcing?
Crowdsourcing, at its core, involves leveraging the power of the crowd to accomplish tasks, generate ideas, or solve problems. This approach taps into the collective intelligence and resources of a large group of people, often via the internet, to achieve a common goal.
Benefits of Crowdsourcing:
- Cost-Effective: Crowdsourcing can significantly reduce costs by distributing tasks across a large group of individuals, often volunteers or freelancers.
- Diverse Perspectives: By involving a wide range of participants, crowdsourcing brings in varied viewpoints and innovative solutions that might not emerge from a traditional, in-house team.
- Speed and Scalability: Tasks can be completed more quickly as they are handled by numerous contributors simultaneously, and the approach can easily scale up to accommodate larger projects.
Examples of Crowdsourcing:
- Product Design and Development: Companies like Lego and Starbucks invite their customers to submit ideas for new products or improvements, fostering a sense of community and loyalty.
- Content Creation: Wikipedia, one of the largest and most popular sources of information, relies on crowdsourcing for its vast array of articles.
- Problem-Solving: Platforms like InnoCentive connect organizations with a global network of problem-solvers who tackle scientific and technical challenges.
AI Data Labeling: The Backbone of Machine Learning
AI data labeling is the process of tagging or annotating data to train machine learning models. This step is crucial because the accuracy and efficiency of AI systems heavily depend on the quality of the labeled data they are trained on.
Importance of AI Data Labeling:
- Improved Accuracy: High-quality labeled data ensures that machine learning models can make precise predictions and decisions.
- Training Supervision: Labeling provides the necessary supervision for training algorithms, helping them to understand and learn from the data.
- Efficiency in AI Development: With accurately labeled data, AI development becomes more streamlined and efficient, reducing the time and resources needed to build robust models.
Methods of Data Labeling:
- Manual Labeling: Involves human annotators meticulously labeling data. While time-consuming, it ensures high accuracy.
- Automated Labeling: Uses pre-existing models to label new data. This method is faster but might require subsequent human validation.
- Crowdsourced Labeling: Combines human intelligence and scalability by engaging a large group of annotators via crowdsourcing platforms.
E-Learning: Revolutionizing Education
E-learning refers to the use of digital platforms to deliver educational content and facilitate learning outside traditional classroom settings. This method has gained immense popularity due to its flexibility, accessibility, and ability to cater to diverse learning styles.
Advantages of E-Learning:
- Flexibility: Learners can access courses at their convenience, making it easier to balance education with other responsibilities.
- Cost-Effective: E-learning often reduces the costs associated with traditional education, such as commuting, textbooks, and accommodation.
- Wide Reach: Online courses can reach a global audience, providing opportunities for people who might not have access to quality education otherwise.
Applications of E-Learning:
- Corporate Training: Companies use e-learning to train employees on new tools, policies, and skills, ensuring consistent knowledge dissemination.
- Higher Education: Universities offer online degrees and courses, allowing students to learn from prestigious institutions regardless of their location.
- Skill Development: Platforms like Coursera and Udemy provide courses on a wide range of topics, helping individuals upskill or reskill to stay competitive in the job market.
Integrating Crowdsourcing, AI Data Labeling, and E-Learning
Combining these three elements can lead to groundbreaking advancements. For instance, crowdsourcing can enhance AI data labeling by providing a large pool of annotators, while e-learning platforms can utilize AI to personalize learning experiences based on user data.
Conclusion:
- Crowdsourcing leverages the collective power of the crowd for innovative solutions.
- AI data labeling is essential for training accurate and efficient machine learning models.
- E-learning offers flexible, cost-effective, and far-reaching educational opportunities.
By understanding and utilizing these concepts, businesses and individuals can unlock new potentials and drive innovation in various fields. Whether you're looking to solve complex problems, train advanced AI models, or provide accessible education, integrating crowdsourcing, AI data labeling, and e-learning can pave the way for success.